
Orthogonal projection-based regularization for efficient model augmentation
This blog post delves into the challenges and solutions associated with deep-learning-based nonlinear system identification models. We explore a proposed solution that integrates prior physical knowledge into the model structure, combining physics-based modeling and deep-learning-based identification. We also discuss an orthogonal projection-based regularization technique that enhances parameter learning, convergence, and model accuracy. The blog further explores the optimization of an augmented model using the SUBNET method, the benefits of the T-step ahead prediction cost, and the importance of input-output normalization. We also touch on the problem of non-unique parametrization and a proposed solution using an orthogonality-promoting term in the cost function.
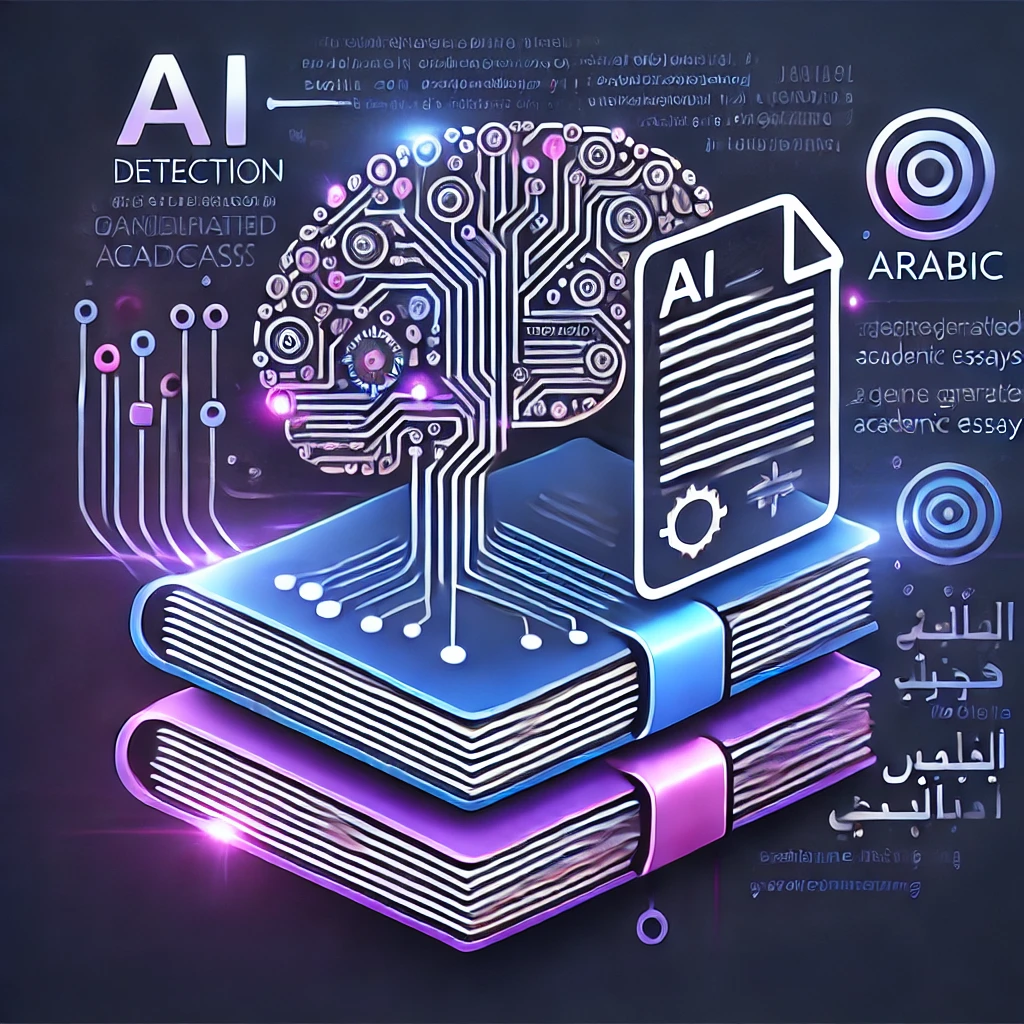
IntegrityAI at GenAI Detection Task 2: Detecting Machine-Generated Academic Essays in English and Arabic Using ELECTRA and Stylometry
In this blog, we delve into the fascinating world of machine learning, focusing on the detection of machine-generated academic essays. We explore the groundbreaking research by Mohammad AL-Smadi from Qatar University, who utilized pre-trained transformer-based models to detect English and Arabic essays. The models, ELECTRA for English and AraELECTRA for Arabic, achieved impressive results with an F1-score of 99.7% and 98.4% respectively. We'll break down the technical aspects of these models, discuss their significance, and provide practical guidance on how to apply these technologies in your own projects.
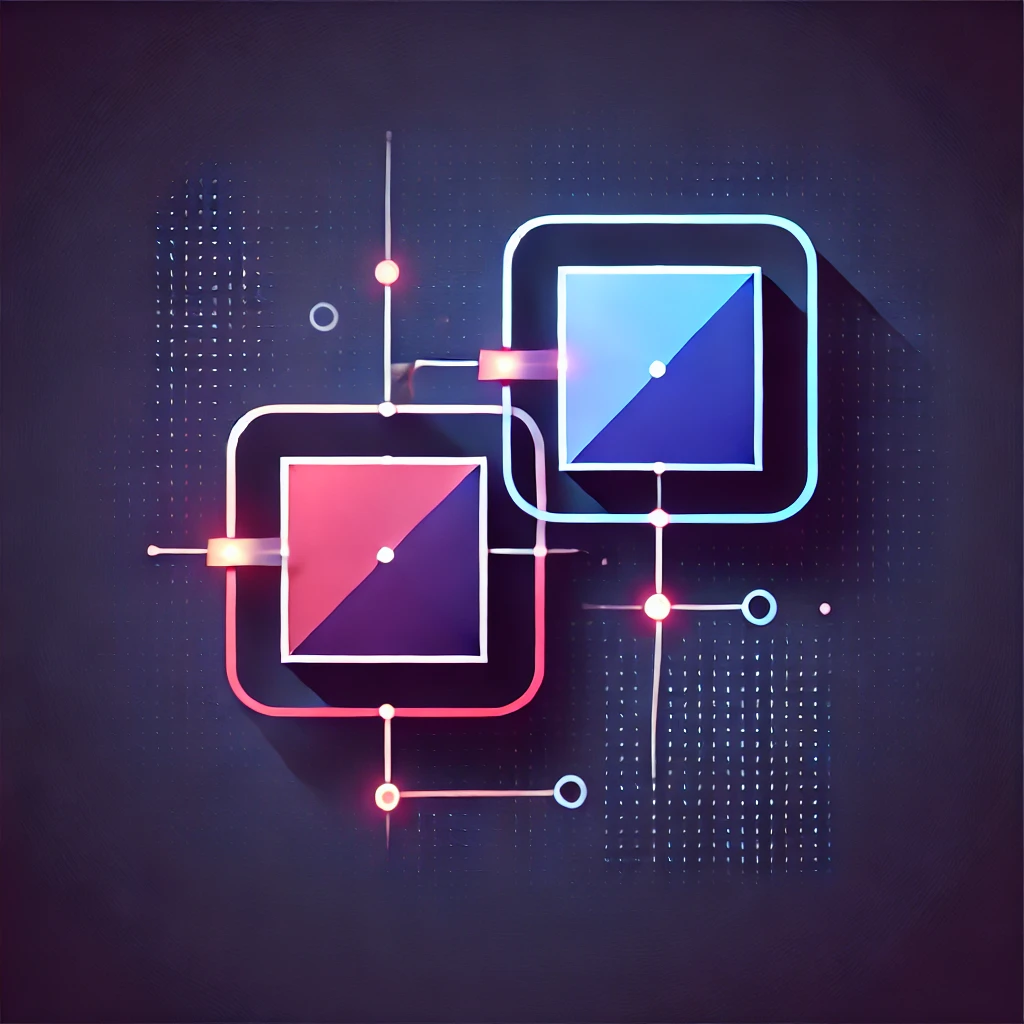
Improving Zero-Shot Object-Level Change Detection by Incorporating Visual Correspondence
In this blog post, we delve into a novel method for improving object-level change detection between two images. We address three major limitations in current approaches: unreported false positives, lack of correspondence, and poor zero-shot generalization across different domains. The proposed method leverages change correspondences during training to enhance change detection accuracy and minimize false positives. We'll explore the scientific article that discusses this method, its key features, and its potential impact on the field of machine learning.
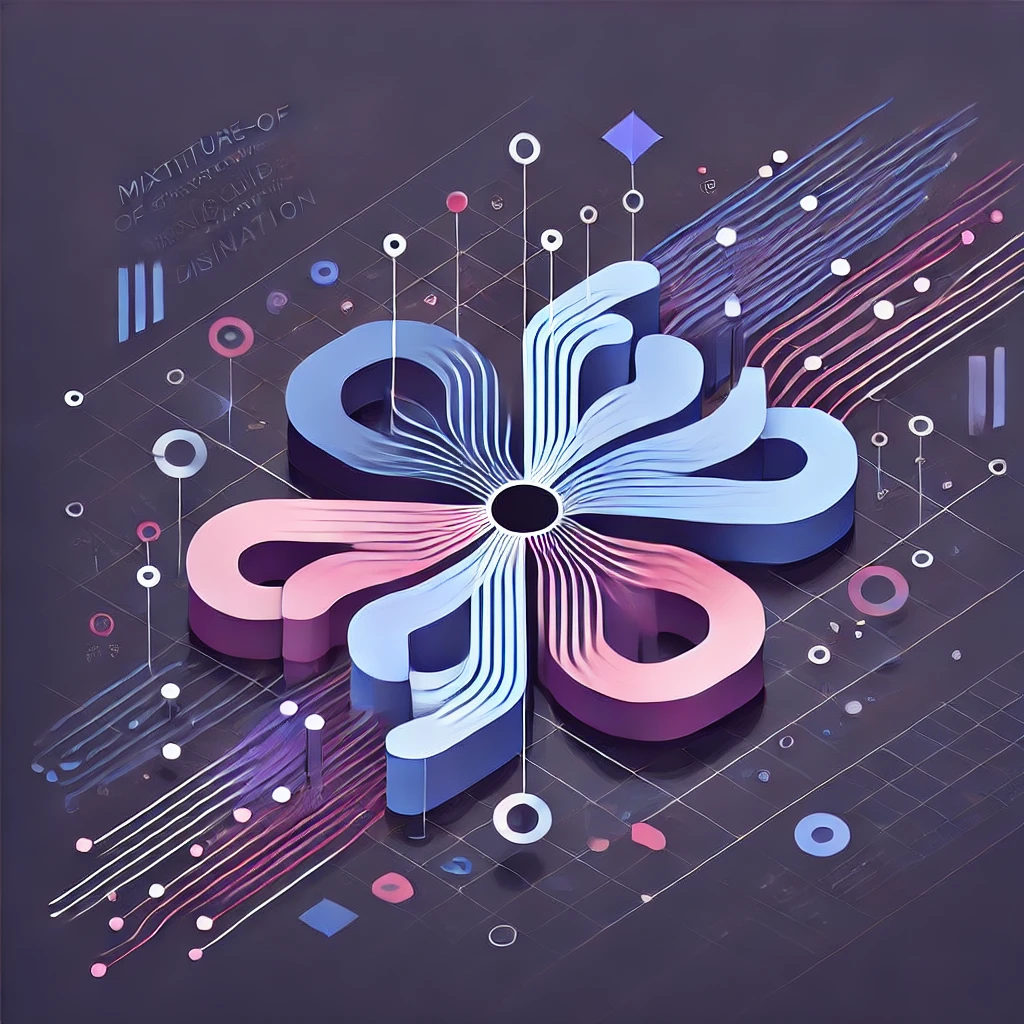
MoVE-KD: Knowledge Distillation for VLMs with Mixture of Visual Encoders
In this blog, we will explore the Mixture-of-Visual-Encoder Knowledge Distillation (MoVE-KD), a novel framework designed to enhance the performance of vision-language models (VLMs). MoVE-KD distills the unique strengths of multiple visual encoders into a single, efficient model, overcoming the computational costs and complexity of incorporating multiple encoders into a single VLM. We'll delve into the technical aspects of MoVE-KD, its historical development, and its potential impact on the field. We'll also provide practical guidance on how to apply this technology in your own projects.
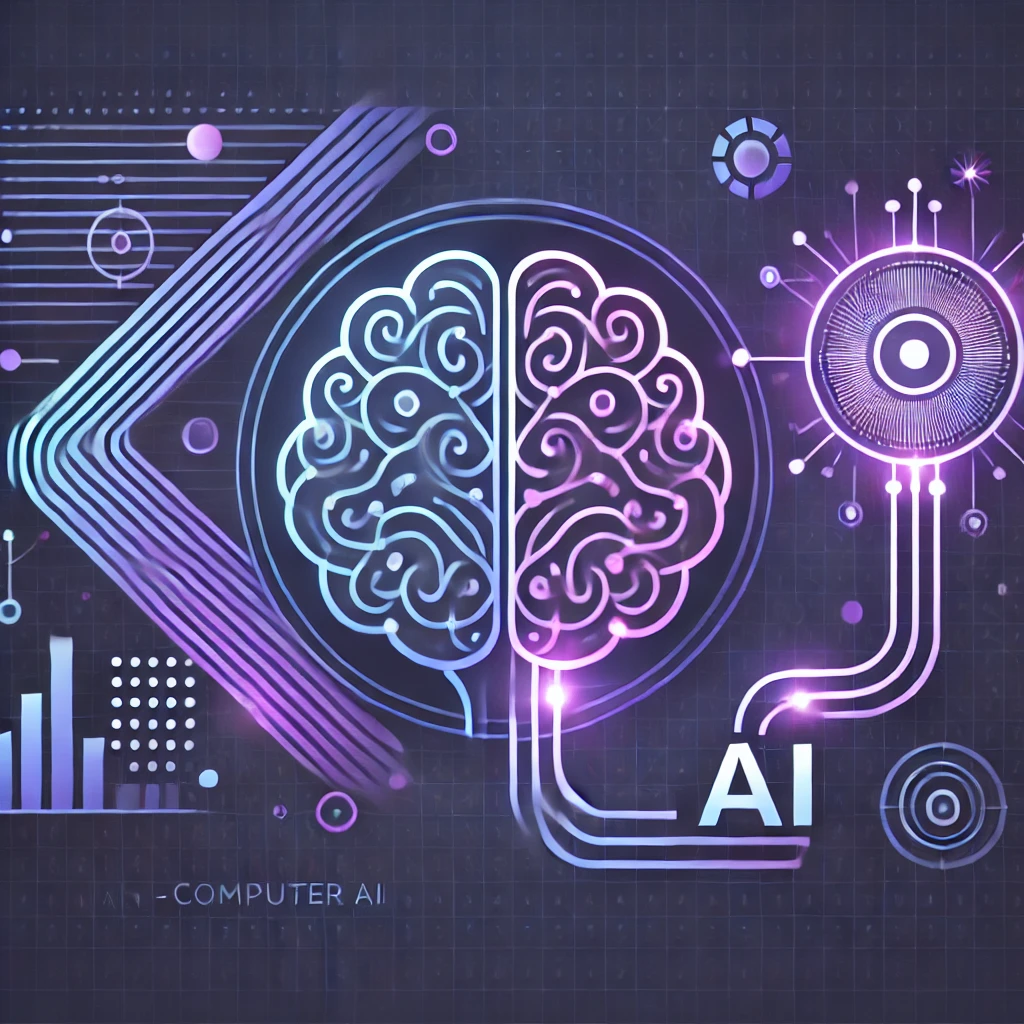
Human-AI Teaming Using Large Language Models: Boosting Brain-Computer Interfacing (BCI) and Brain Research
This blog post explores the fascinating intersection of artificial intelligence (AI) and brain-computer interfacing (BCI), with a particular focus on the role of large language models (LLMs) in enhancing brain research. We delve into the concept of human-AI collaboration, the Janusian design principles that underpin this approach, and the innovative ChatBCI toolbox that brings these principles to life. We also discuss the potential of lightweight neural networks, the importance of dataset diversity, and the need for improved model interpretability. Finally, we look at the broader implications of these advancements, including the potential for 'brain-grokking AI' to revolutionize our understanding of human cognition and mental health interventions.

Efficient LLM Inference with Activation Checkpointing and Hybrid Caching
In the realm of machine learning, large language models (LLMs) are becoming increasingly critical. However, their growing model sizes require significant GPU memory capacity, leading to high costs. This blog post delves into the challenges of key-value (KV) cache management and batched LLM inference, and introduces HybridServe, a system designed to enhance the efficiency of LLMs. HybridServe uses a hybrid caching strategy, employing both KV cache and Activation cache to optimize performance. The system also employs a two-step allocation policy to balance KV and ACT blocks in host memory, and a dynamic mini-batch formation to balance KV and activation within a single request. The result is a significant improvement in GPU utilization and a reduction in excessive computations.
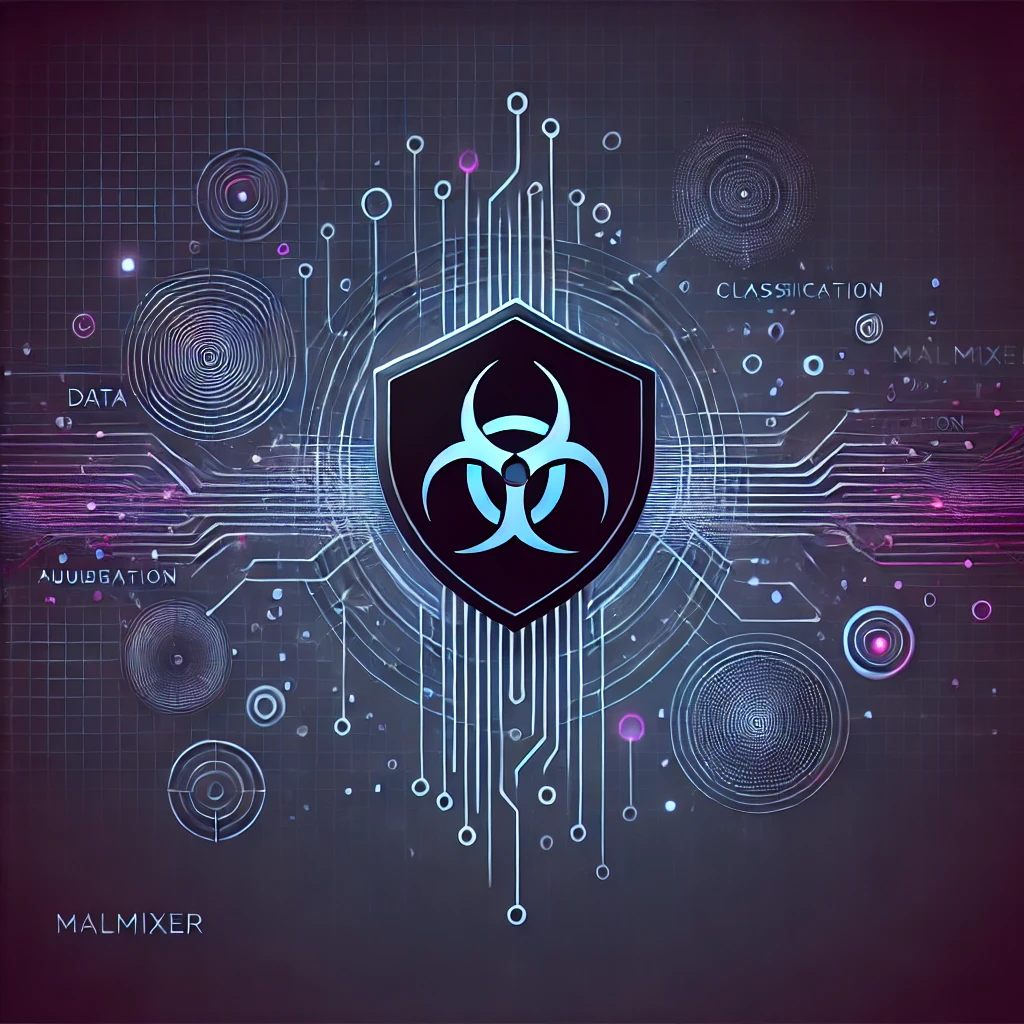
MalMixer: Few-Shot Malware Classification with Retrieval-Augmented Semi-Supervised Learning
This blog post delves into the workings of MALMIXER, an innovative malware family classifier that leverages semi-supervised learning to classify malware with limited training data. It uses a novel similarity-and-retrieval-based augmentation technique to generate synthetic data and aligns this data to mimic ground-truth family distributions. The blog will explore the model's architecture, its significance in the field of malware detection, and its potential impact on the industry. It will also provide a technical analysis of the model and practical guidance on its application.

Filter or Compensate: Towards Invariant Representation from Distribution Shift for Anomaly Detection
In this blog, we delve into the challenges of Anomaly Detection (AD) methods in handling real-world data that exhibits distribution shift. We introduce Filter or Compensate (FiCo), a system designed to address this issue by compensating distribution-specific information and filtering abnormal information to capture distribution-invariant normality. We explore how FiCo outperforms existing methods, even in in-distribution scenarios compared to Reverse Distillation-based methods. We also discuss tackling the "normality forgetting" issue in Out-of-Distribution (OOD) generalization, and the introduction of a novel scientific method, the DiIFi module, used in network training. Lastly, we present the results of the FiCo system performance on different datasets.

CAS-ViT: Convolutional Additive Self-attention Vision Transformers for Efficient Mobile Applications
This blog post explores the Convolutional Additive Self-attention Vision Transformers (CAS-ViT), a novel approach to neural networks that optimizes efficiency and performance for mobile applications. We delve into the unique architecture of CAS-ViT, its development, and its impact on the field of machine learning. We also offer a technical analysis of the model, practical guidance on its application, and a comprehensive FAQ section for further clarification. By the end of this read, you'll have a deep understanding of CAS-ViT and its potential to revolutionize mobile applications.
Simplify ML development
and scale with ease
Join the researchers and engineers who use Modlee
Join us in shaping the AI era
MODLEE is designed and maintained for developers, by developers passionate about evolving the state of the art of AI innovation and research.